Decisions are the critical factors that define the success or failure of an organisation. While intuition and experience remain vital components of decision-making, modern decisions increasingly rely on data. In today’s complex business ecosystem, where new ventures emerge constantly, data serves as a guiding light.
To stand out and ensure your business outperforms competitors, you need data to validate your intuition and expertise or to correct you when you’re drifting from reality. However, with the immense volumes of data available today, effectively utilising it can be a daunting task. This is where intelligent decisioning systems come into play. These systems not only process data but also interpret it, predict outcomes, and recommend optimal courses of action, ultimately helping you drive business revenue. Best of all, they do this in real time.
The Need for Intelligent Decisioning Systems
Businesses today are inundated with data. And with almost every business relying on data the data consumption and creation is increasing. With approximately 402.74 million terabytes of data generated daily, the total amount of data created, consumed, and stored globally is projected to exceed 394 zettabytes by 2028. While this data holds immense potential, its sheer volume can be overwhelming. Without a structured approach, companies risk missing crucial insights hidden within their datasets.
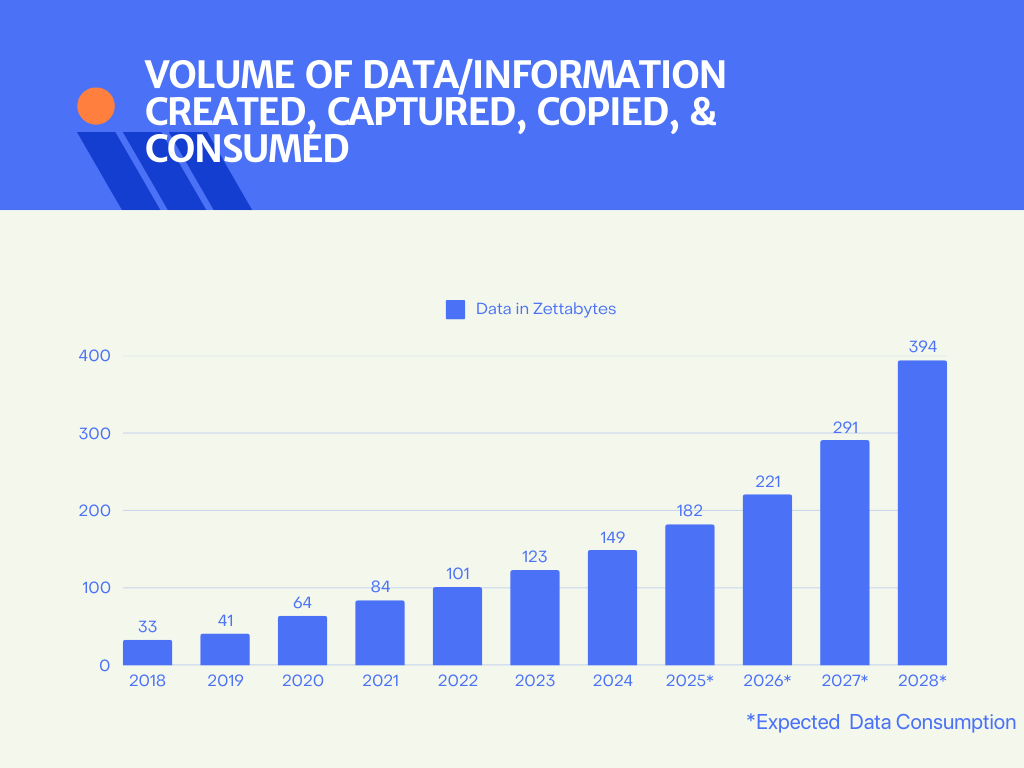
Take the retail sector as an example. Retailers collect vast amounts of data on customer preferences, buying patterns, and seasonal trends. Analysing all this information manually or even with basic tools is not only time-consuming but also prone to errors. Yet, a wrong pricing strategy or misjudging inventory can lead to significant losses. Intelligent decisioning systems step in to bridge this gap, enabling businesses to leverage data effectively and make well-informed decisions.
How Intelligent Decisioning Systems Bridge the Gap Between Data and Decisions
Intelligent decisioning systems bridge the gap by transforming raw, unstructured data into meaningful insights that decision-makers can act upon. Here are the key steps involved:
- Data Aggregation: These systems pull data from various sources—internal databases, external APIs, IoT devices, and even alternative data sources like social media platforms. By consolidating data into a single framework, they eliminate silos and provide a holistic view of the business.
- Data Cleaning and Preparation: Raw data is often incomplete or inconsistent. Intelligent systems automatically clean, normalise, and structure data to ensure it’s accurate and ready for analysis.
- Advanced Analytics: Using techniques like machine learning, predictive analytics, and natural language processing, these systems uncover patterns, trends, and correlations that might not be apparent to the human eye.
- Real-Time Processing: Unlike traditional methods that analyse historical data, intelligent systems process information in real time. This enables businesses to respond quickly to changes in market conditions, customer behaviour, or operational issues.
- Actionable Insights and Recommendations: The intelligent decisioning system generates clear, actionable insights tailored to specific business needs. For instance, it might recommend adjusting inventory levels based on predicted demand or suggesting optimal pricing strategies.
- Decision Support: These systems don’t replace human judgment—they enhance it. By presenting multiple scenarios and possible outcomes, they empower decision-makers to evaluate options and make well-informed, data-driven decisions.
By automating these steps, intelligent decisioning systems save time, reduce errors, and ensure that decisions are grounded in data rather than assumptions.
Empowering Decision-Makers Across Industries
The value of intelligent decisioning systems extends across different sectors. Here are a few examples of how these systems are transforming industries:
Medical: AI in medicine goes beyond just managing patient data—it is now actively assisting physicians in clinical practice. Researchers at Google Inc. and partner institutions have demonstrated that an AI system, trained on thousands of images, can match physicians in diagnosing referable diabetic retinopathy (DR) with high sensitivity and specificity.
Moreover, the system has identified previously unrecognised links between image patterns in fundus photographs and cardiovascular risk factors, showcasing AI’s growing potential in medical diagnostics.
Supply Chain: Intelligent decisioning systems revolutionise supply chain management by integrating real-time data from various sources, such as GPS tracking, weather forecasts, and customer demand trends.
For example, e-commerce giants like Amazon use AI-driven decisioning to dynamically adjust delivery routes, minimising delays due to traffic or weather conditions. These systems analyse historical sales patterns and external factors like market trends to predict demand fluctuations, helping businesses avoid overstocking or stockouts.
Marketing: Marketing has shifted from mass advertising to hyper-personalised targeting, thanks to intelligent decisioning systems. These systems analyse customer demographics, browsing history, purchase patterns, and engagement metrics to predict what a customer is most likely to buy and when. For instance, companies like Netflix and Amazon use predictive analytics to recommend content based on users’ past viewings.
Balancing Automation with Human Judgment
While intelligent decisioning systems offer powerful capabilities, they are not a replacement for human expertise. Instead, they serve as an augmentation tool, providing decision-makers with the insights they need to make informed choices. Think of it as a partnership between humans and technology—one where the strengths of both are leveraged to achieve optimal outcomes.
A real-world example of this balance can be seen in manufacturing. Predictive maintenance systems use sensors and data analytics to identify potential equipment failures before they occur. While the system can alert the team about a specific issue, it’s ultimately up to human engineers to decide the best course of action based on operational priorities and cost considerations.
Final Thoughts
In today’s fast-paced business environment, the ability to bridge the gap between data and decisions is not just an advantage—it’s a necessity. Intelligent decisioning systems provide the tools and insights needed to navigate complexities, seize opportunities, and mitigate risks. However, their success ultimately depends on how businesses integrate them into their workflows and how well they balance technology with human judgment.
Corestrat’s ID.ai is an intelligent decisioning system that is capable of analysing vast amounts of data and helping organisations to make informed decisions at scale for improved productivity and profitability. Users can upload data from multiple sources, select target variables, and auto-grow decision trees to obtain recommendations and predictions using ID.ai.
As we move forward, one thing is clear: businesses that harness the power of intelligent decisioning systems will not only make better decisions but will also position themselves as leaders in their industries. The question is, are you ready to make that leap?