Model.ai: No Code AI Model Builder
Build and Deploy ML Models in Minutes
Drive better business decisions by leveraging Ecosystem Risk Score (ERS) to unlock the true potential of your non-traditional user data.
What is Model.ai?
Corestrat’s Model.ai is a no-code, enterprise-ready platform that allows you to build and deploy classification and/or regression predictive models in a few clicks.
Model.ai helps enterprise users to get closer to smarter business decisions by capturing actionable and hidden patterns in the data.
Model.ai automates a large part of repetitive machine learning steps to ease the tasks of data scientists & non data scientists, thereby enabling enterprises swiftly adopting ML solutions and allowing them to focus on more complex issues.
Leveraging Alternative Data for Credit Decisioning
Get the full picture of your borrowers’ financial health by combining the predictive power of Model.ai with your ecosystem usage data through Ecosystem Risk Score (ERS).
Ecosystem Risk Score and Predictive Intelligence for 3x Credit Approvals
Unlock the true potential of your ecosystem usage data through predictive intelligence to drive better business decisions.
Ecosystem Risk Score, powered by Model.ai, enables fintechs and digital banks increase lending volume by over 30% through risk-informed credit limit and pricing decisions.
ERS offers a unique competitive edge providing differentiated insights leveraging existing ecosystem data to drive better and informed decisions.
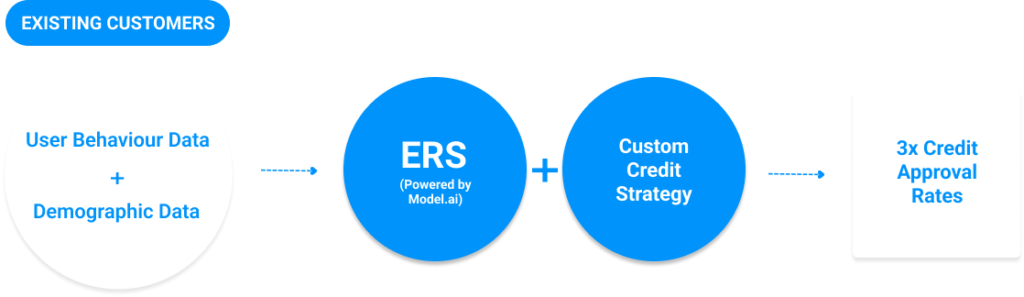
*Dynamic learning to optimise approval rate (enabled with Federated Learning Models)
Ready-To-Use ML Model Builder With Advanced Features
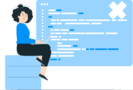
No-Code Model Building
No training, no programming, no formulas platform that enables both, data scientists and non-data scientists, to build predictive models in a few clicks. It offers simple, secure and flexible products with an easy-to-use graphical interface to build predictive models.

Continuous Learning
Model.ai is AutoML-enabled, a technology that automates building high-performing models, and continuously learns from previous data to generate better outcomes. With AutoML, developers with little prior knowledge of ML can train models based on specific business needs and goals.
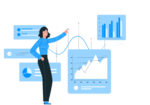
Centralised Dashboard
Get immediate visualisation of your model output including model variable importance, output explainability reports, model monitoring reports,
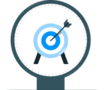
Transparency and Accuracy
Build predictive models with consistency, and minimised inaccuracies caused by bias or human mistakes. With extensive automation and transparency throughout the machine learning lifecycle, Model.ai enables data science teams with the accuracy, speed and confidence required to build and deploy auditable models.
Build Your Own ML Model in Just a Few Steps​

Upload data
Upload data files in CSV or XLS format

Choose target
Select the target variable which you want to measure and let Model.ai do its magic.

Model output
Evaluate XG Boost, Logistic Regression and Random Forest models with the help of multiple statistical measures.
ML-Powered Lending Automation Platform That Grows With You
Advanced capabilities of Model.ai reduce data scientists’ time spent on repetitive tasks, enabling them to focus on more important matters.
Binary classification
Supervised learning algorithm that categorises new observations into one of two classes
Model documentation
Download the business-level document of the entire modeling process in one click
Variable binning
Group variables manually and automatically to create a monotonic trend using WOE and Information Value for all the variables
Feature Importance
Determining the irrelevant/insignificant variables and removing them automatically
3 best models
Build a model choosing from Random Forest, XG Boost, and Logistic Regression to predict your outcome
Label encoding
The platform automatically converts labels into a numeric, machine-readable form
Control variable reduction settings
Users can reset the default reduction settings as per their needs
Let ML-Powered Models Guide Your Key Business Decisions
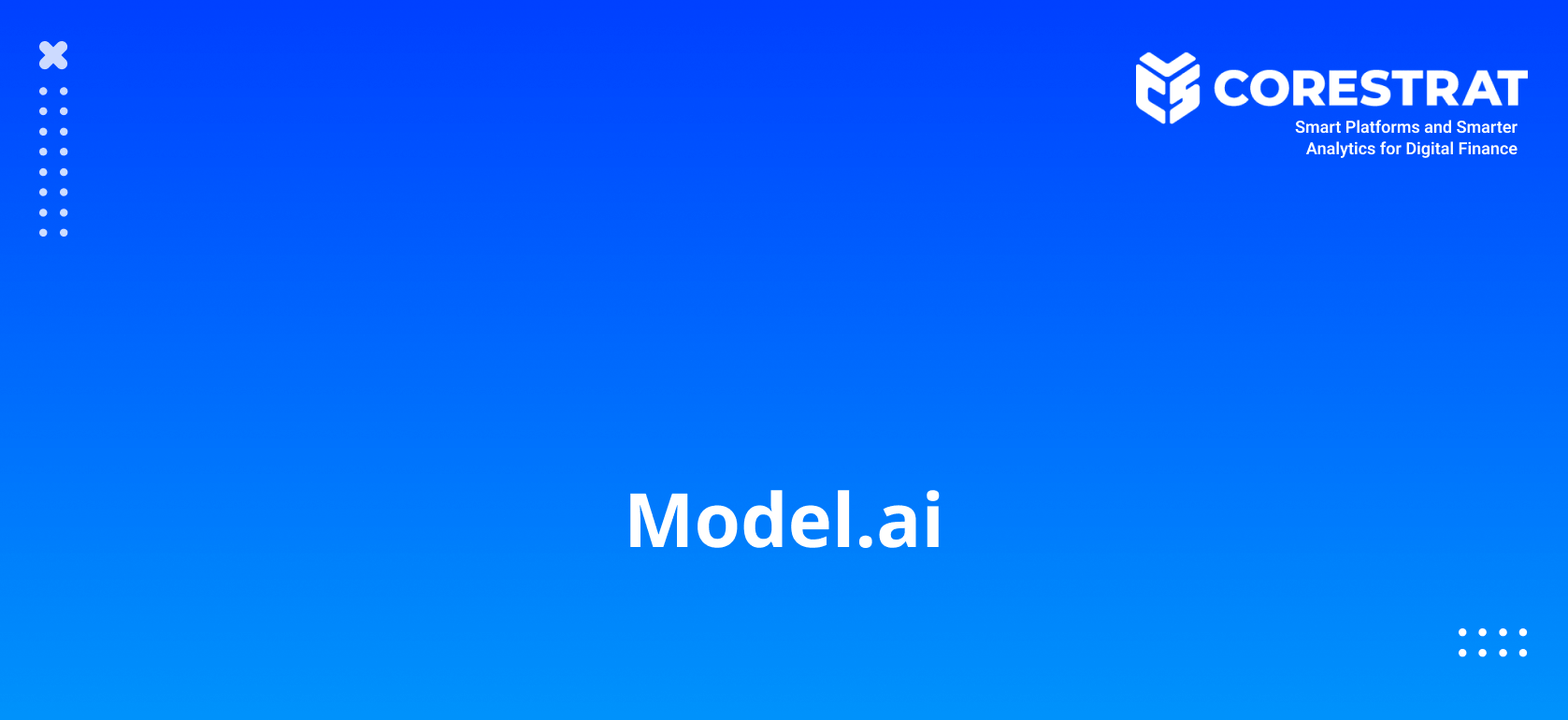
What’s In It For You?
Increase customer retention by predicting and managing customer churn
Predict and manage credit risk with the right AutoML models
Increase productivity and democratise ML tools
Bridge gaps between data scientists and ML problems
Save costs and resources and earn a greater ROI
One Platform. Multiple Use Cases.
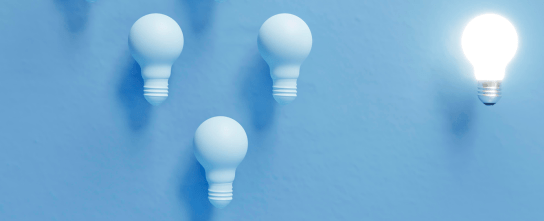
By Solutions
- Application scoring
- Customer segmentation
- Credit approvals/ rejections
- Sales/revenue forecasting
- Fraud detection, and more
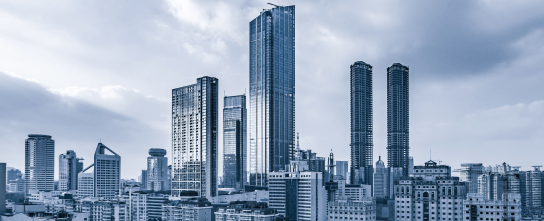
By Industry
- Banking
- Financial Services
- Healthcare
- Manufacturing & FMCG
- Supply chain & logistics, etc.
Discover Corestrat's Complete Product Suite
Why Choose Corestrat?

Talented Team with Decades of Global Experience in Multiple Business Sectors.

AI Powered Digital Transformation with Expanded Control and Visibility.

Intelligent Automation Smart Platforms and Analytics-Based Advisory.

Intuitive Technology Architecture & Streamlined Business Processes.
FAQ's
A predictive model is a statistical or machine learning model that is trained on historical data to make predictions or forecasts about future outcomes. It uses the patterns and relationships in the data to infer or estimate unknown values.
Any AI-powered tool follows the following set of principles to build a predictive model:
- Define the problem and desired outcome.
- Collect relevant data.
- Preprocess the data by cleaning, handling missing values, and scaling.
- Select or engineer important features.
- Choose an appropriate model based on the problem and data.
- Train the model on a training set.
- Evaluate the model’s performance using appropriate metrics.
- Refine the model by adjusting parameters or trying different algorithms.
- Deploy the model to make predictions on new data.
- Monitor and maintain the model’s performance, updating it as needed.
A predictive model builder like Model.ai is valuable because it enables accurate forecasting, supports decision-making, provides customer insights, aids in risk assessment and fraud detection, optimises processes, and contributes to research and innovation. It empowers organisations and individuals to leverage data and make informed, data-driven decisions.
There are various algorithms used in predictive modelling, including Linear Regression, Logistic Regression, Decision Trees, Random Forests, Support Vector Machines (SVM), Gradient Boosting, Neural Networks, and more. The choice of algorithm depends on factors such as the type of problem, the data characteristics, and the desired interpretability or accuracy.
Model.ai uses XGBoost, Random Forest and Logistic Regression algorithm to build predictive models for its users.
Common challenges in predictive modelling include obtaining high-quality and relevant data, dealing with missing or noisy data, selecting appropriate features, choosing the right algorithm, handling overfitting or underfitting, interpreting the model’s predictions, and adapting to changing data patterns over time.
Let's Get Started!
Our experts are happy to connect with you to explore how we can help your enterprise innovate.