In the ever-evolving landscape of finance, managing credit risk has remained a paramount concern for banks, financial institutions, and lenders around the world. Credit risk, the potential for borrowers to default on their obligations, poses significant challenges to the stability and profitability of these institutions. The ability to assess and mitigate credit risk is not only a critical factor in maintaining a healthy financial portfolio but also a crucial component of regulatory compliance.
Traditional methods of credit risk assessment have relied on historical data, financial ratios, and statistical models. While these methods have proven effective to some extent, they often fall short of capturing the complexities of today’s dynamic financial markets.
Enter Artificial Intelligence (AI) and Machine Learning (ML), two transformative technologies that have revolutionised the way financial institutions approach credit risk management. According to Allied Market Research, the AI market within the banking sector was valued at $3.88 billion in 2020, and it is projected to surge to an impressive $64.03 billion by the year 2030. Given that credit risk management stands as a top priority for any fintech, discussing the significance of AI and ML in this context is entirely justified.
AI and ML algorithms have the capacity to process vast amounts of data, recognise intricate patterns, and make real-time decisions with remarkable accuracy. In the realm of credit risk management, these technologies have unlocked new opportunities for enhancing decision-making processes, improving loan origination, and reducing credit losses. By harnessing the power of AI and ML, financial institutions can now delve deeper into customer behaviour, extract valuable insights, and predict creditworthiness more effectively than ever before.
This blog serves as an introduction to the intersection of Credit Risk Management and AI/ML, offering a comprehensive exploration of how these technologies are reshaping the landscape of financial risk assessment.
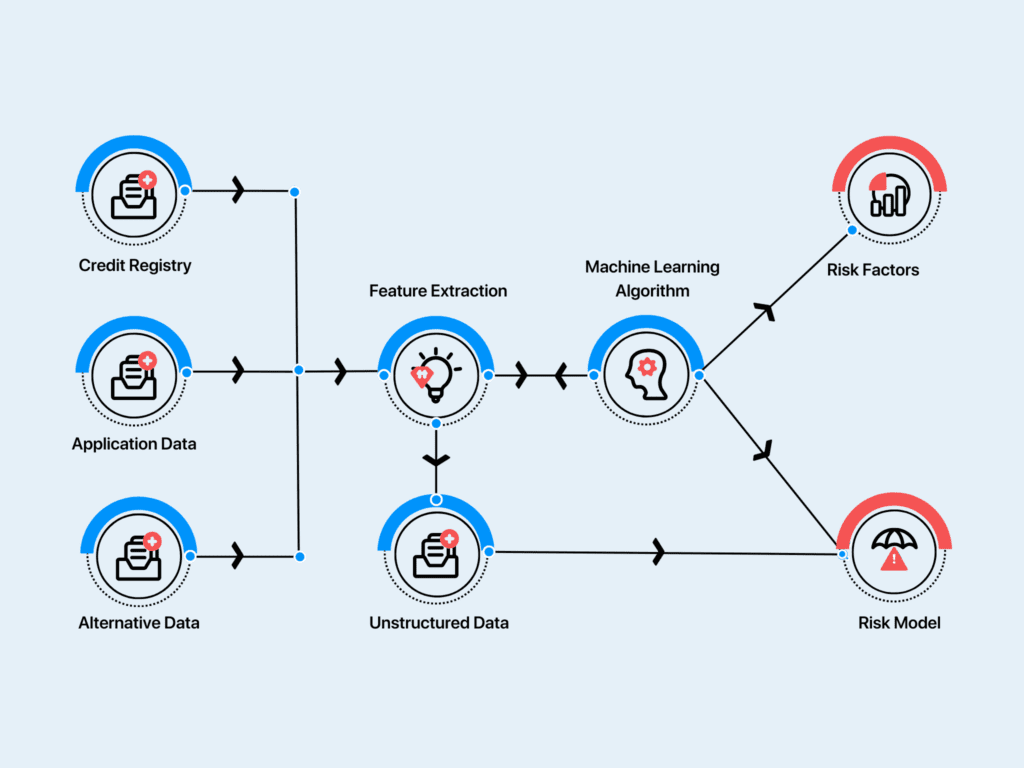
How to implement AI and ML in credit risk management?
Implementing AI and ML in fintech credit risk management is a complex yet essential process. It starts with collecting diverse data and cleaning it meticulously to ensure data quality. Feature engineering helps identify influential variables and prepares them for model training. Model selection involves choosing suitable algorithms, potentially employing ensemble methods for accuracy. Training models on split datasets teaches them to recognise credit risk patterns.
Evaluation metrics validate their performance and ensure they don’t overfit or underfit. Hyperparameter tuning optimises the models. Deployment integrates these models into the fintech’s systems, allowing real-time lending decisions. Continuous monitoring and maintenance are vital for model performance. Ethical and regulatory considerations address bias, fairness, and compliance with data privacy laws. Transparency and explainability build trust, while security measures protect sensitive data. Finally, scaling and integration ensure adaptability as the fintech grows. A multidisciplinary team and ongoing commitment are crucial for a successful implementation that enhances credit risk management while mitigating risks.
5 benefits of AI and ML in fintech credit risk management
Artificial Intelligence (AI) and Machine Learning (ML) offer several significant benefits in fintech credit risk management:
Improved Accuracy in Credit Scoring
AI and ML algorithms possess the remarkable capability to delve into a wide spectrum of data sources, extending beyond traditional metrics to encompass unconventional ones such as social media behaviour and online transactions. This breadth of data analysis leads to a level of comprehensiveness that greatly enhances the accuracy and predictiveness of credit scores.
By embracing this holistic approach, fintech companies gain the capacity to make more astute judgments regarding an applicant’s creditworthiness. This, in turn, translates into a substantial reduction in the risk of defaults – a pivotal advantage in the financial sector where minimising credit risk is of paramount importance.
The ability to consider a richer tapestry of factors empowers fintechs to craft more nuanced lending decisions, ultimately fostering more robust and reliable credit risk management practices.
Faster Decision-Making
The infusion of AI and ML into the lending landscape has ushered in a new era of expeditious decision-making. Through the deployment of automated loan approval systems, fintech companies have harnessed the power to dramatically accelerate the lending process. Gone are the days of prolonged waiting periods and cumbersome paperwork; today, fintech can swiftly and efficiently assess loan applications in real-time, often completing the evaluation within a matter of seconds.
This not only revolutionises the way lending operates but also delivers an unparalleled user experience. Customers can now access credit promptly, precisely when they require it, removing the hurdles that traditionally impeded timely financial assistance. This remarkable acceleration in decision-making is emblematic of the transformative impact AI and ML have had on the fintech industry, making financial services more accessible and responsive than ever before.
Reduced Operational Costs
The adoption of AI and ML technologies in credit risk assessment brings forth a compelling financial advantage: reduced operational costs. Through the automation of critical credit risk evaluation processes, fintech companies can achieve significant savings in their operational budgets. One of the primary avenues for cost reduction lies in minimising the necessity for manual intervention in the loan approval process.
Traditional methods often required a considerable workforce to manually review and assess loan applications, a labour-intensive and time-consuming endeavour. By contrast, AI and ML-driven systems can swiftly and accurately analyse vast datasets, swiftly sifting through a multitude of variables to gauge creditworthiness. This not only expedites the decision-making process but also markedly diminishes the need for a large staff to handle these tasks, ultimately leading to considerable reductions in staffing costs.
Moreover, the automation of credit risk assessment significantly mitigates the potential for human errors, which can be costly in both financial and reputational terms. AI and ML models are designed to operate with a high degree of precision and consistency, thereby reducing the risk of costly mistakes that may arise from manual data entry or subjective decision-making. This enhanced accuracy contributes further to cost savings, as it can save up to 20% in operational costs as errors and their associated consequences can be reduced.
In summary, the incorporation of AI and ML into credit risk management not only augments the efficiency and accuracy of processes but also yields substantial cost savings by streamlining operations, reducing staffing needs, and minimising the likelihood of costly errors. This financial benefit positions fintech companies to allocate resources more strategically and enhance their competitive edge in the rapidly evolving financial services landscape.
Enhanced Fraud Detection
The significance of AI and ML in fraud detection within the lending sector cannot be overstated. In recent times, these technologies have emerged as pivotal tools in the relentless battle against fraudulent activities. AI and ML for fraud detection in lending have become significant these days as they can detect unusual patterns and anomalies in transaction data, helping fintech identify and prevent fraudulent activities. This capacity for continuous, vigilant monitoring of transactions and user behaviour represents a formidable deterrent to would-be fraudsters, as it enables real-time alerts and interventions.
According to a study, the implementation of artificial intelligence (AI) exclusively for the purpose of fraud detection has resulted in remarkable cost savings for the bank, amounting to an impressive $150 million annually. This monumental financial benefit underscores the unparalleled value that AI brings to a financial institution’s endeavours in combatting fraudulent activities. Beyond the financial aspect, the profound implications extend to safeguarding the institution’s reputation, maintaining customer trust, and upholding the integrity of the financial system as a whole. In a world where cyber threats and financial fraud are ever-evolving, the role of AI and ML in bolstering security and minimizing risks cannot be overstated.
Optimised Portfolio Management
AI and ML can help fintechs optimise their loan portfolios. These technologies can segment loan portfolios based on risk profiles and automate risk monitoring. Financial institutions using AI and ML for portfolio optimisation have seen a 12% increase in overall portfolio performance. By proactively identifying high-risk loans and taking appropriate actions, fintechs can minimise losses and improve the overall performance of their lending portfolios.
These benefits not only make fintech credit risk management more efficient and cost-effective but also enhance the accuracy of lending decisions, reduce risks, and improve the overall customer experience. As a result, fintech companies are better positioned to compete in the market, attract investors, and build trust with their customers.
Conclusion
Today, within the fintech industry, artificial intelligence (AI) and machine learning (ML) serve as the fundamental pillars underpinning credit risk management. These cutting-edge technologies have empowered fintech companies with the capability to analyse vast amounts of data, enabling them to tackle fraudulent activities seamlessly and enhance risk management.
AI and ML not only bolster the efficiency and cost-effectiveness of credit risk management in fintech but also elevate the precision of lending decisions, mitigate risks, and elevate the overall customer experience.
At Corestrat, our suite of products, known as the Intelligent Decision Studio, harnesses the power of AI and ML. These tools enable fintech and lending institutions to wield data effectively in their risk management processes, enabling data-driven decision-making. Consequently, fintech firms that integrate our suite of products are better positioned to thrive in the market, attract investors, and foster trust among their customers.