The lending industry has witnessed a significant transformation in today’s digital era. The advent of online lending platforms and the proliferation of digital transactions have provided convenience and accessibility to borrowers. However, this shift has also exposed lenders to an increased risk of fraud.
Banks worldwide encountered a substantial financial impact from fraudulent activities in 2021, resulting in losses amounting to $328 billion. With financial institutions having to spend a minimum of $4 for every dollar consumer losses due to fraud, fraud prevention has become a great deal for these institutions in terms of money and reputation.
To combat this challenge, the integration of Artificial Intelligence (AI) and Machine Learning (ML) techniques has become crucial for fraud detection in lending. By leveraging advanced algorithms, lenders can effectively identify suspicious activities, mitigate risks, and ensure the integrity of their lending operations.
The global AI in fraud detection and management market is anticipated to reach a valuation of $10,473.3 million in 2023 and is projected to grow at a compound annual growth rate (CAGR) of 18% to reach $57,146.8 million by 2033. This substantial growth highlights the prominence of AI and ML as significant technologies in the field of fraud detection.
Understanding Fraud in Lending
Fraud in lending refers to any deceptive activity carried out with the intent of obtaining funds or benefits illegally. Fraudsters employ various tactics, including identity theft, loan stacking, falsified documentation, and synthetic identities, to exploit vulnerabilities within the lending process.
Traditional methods of fraud detection, such as manual reviews and rule-based systems, often fall short in identifying complex fraudulent patterns. This is where AI and ML come into play, revolutionising fraud detection and prevention in the lending industry.
The Power of AI and ML in Fraud Detection
AI and ML algorithms have the ability to analyse vast amounts of data in real time, detect patterns, and identify anomalies that are indicative of behaviour. By learning from historical data, these technologies continuously evolve and adapt to new fraud schemes, making them highly effective in mitigating risks. Here are some key ways in which AI and ML contribute to fraud detection in lending:
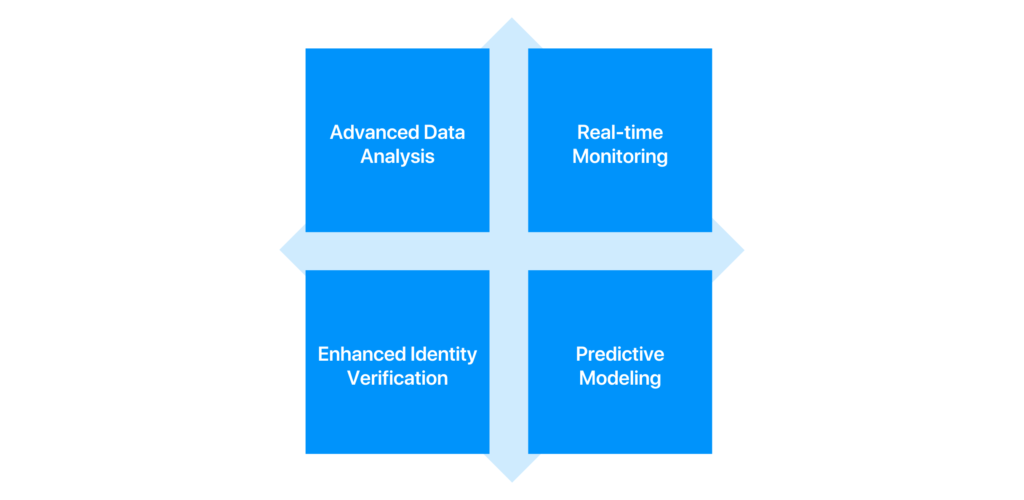
Advanced Data Analysis
AI and ML techniques enable lenders to process and analyse large volumes of data, including borrower information, credit histories, transactional data, and external non-traditional data sources. By applying complex algorithms, these technologies can identify hidden relationships, detect abnormal patterns, and flag suspicious activities that may indicate fraudulent behaviour and help in risk management effectively.
Reports indicate that an optimised machine learning solution, specifically designed for analysing vast volumes of data, has the capability to detect up to 95% of fraudulent activities. By effectively identifying fraud, this solution significantly reduces the expenses associated with manual processes.
AI and ML-based technology, with its remarkable accuracy and efficiency, surpasses conventional methods in identifying fraudulent activities. This advanced technology not only saves valuable time but also offers cost-saving benefits, enabling more effective detection of fraudulent behaviour.
Real-time Monitoring
Since its inception in 1992, real-time monitoring has been instrumental in reducing fraud by approximately 70% in the United States. This advancement has significantly contributed to the safety and security of transactions for various institutions. With the integration of AI-powered real-time monitoring systems, the effectiveness has further improved. These systems possess the ability to promptly identify transaction irregularities by conducting thorough analyses and comparisons with historical data.
AI-powered fraud detection systems provide real-time monitoring capabilities, allowing lenders to identify and respond to potential fraud as it occurs. Automated alerts can be triggered for unusual activities, such as multiple loan applications from the same IP address or sudden changes in behaviour. This proactive approach helps prevent losses and minimises the impact of fraud.
By operating round-the-clock, AI and ML possess the capability to detect any irregularities within a borrower’s loan application promptly. This constant monitoring empowers lending institutions to swiftly identify anomalies and make informed decisions when providing loans.
Enhanced Identity Verification
In 2022, identity fraud caused substantial losses of $43 billion in the United States, impacting more than 40 million US citizens. While identity theft remains a prevalent issue, the fintech industry has witnessed a significant reduction in its impact due to the emergence of AI.Â
AI and ML techniques bolster identity verification processes by incorporating multi-factor authentication and biometric recognition. By leveraging facial recognition, fingerprint scanning, and voice recognition technologies, lenders can ensure that borrowers are who they claim to be, reducing the risk of identity theft and synthetic identity fraud.
Predictive Modeling
Predictive analytics has become the key in making calculated decisions for lending institutions today with many relying on it to minimise the losses while giving out loans. ML algorithms can build predictive models based on historical data, enabling lenders to assess the likelihood of a loan application being fraudulent. These models evaluate a range of factors, including credit scores, employment history, and previous instances of fraud, to generate risk scores. Lenders can use these scores to make informed decisions regarding loan approvals and determine the level of scrutiny required for each application.
Model.ai, developed by Corestrat, is an exceptional predictive model builder. This no-code, enterprise-ready platform empowers users to effortlessly create and deploy classification and regression predictive models with just a few clicks. Lenders can make use of Corestrat’s Ecosystem Risk Score – powered by Corestrat’s Model.ai – a cutting-edge tool that helps you harness the power of alternate data to assess the creditworthiness of the borrower. This cutting-edge solution analyzes a range of non-traditional data related to ecosystem usage, enabling lenders to gain a comprehensive understanding of a borrower’s financial well-being. Consequently, lenders can make more informed and lucrative credit decisions, resulting in improved profitability and preventing fraud.
Conclusion
As the lending industry continues to evolve in the digital era, fraud detection becomes a critical aspect of ensuring the integrity of lending operations. AI and ML technologies provide lenders with powerful tools to combat fraud effectively. By leveraging advanced data analysis, real-time monitoring, enhanced identity verification, and predictive modelling, lenders can mitigate risks and protect themselves and borrowers from fraudulent activities. However, it is important for lenders to navigate the challenges and ethical considerations associated with these technologies to strike the right balance between security, transparency, and customer experience in the lending process.
Â
Presently, Corestrat has established itself as a prominent provider of AI and ML-powered tools, offering valuable assistance to lending institutions in their battle against fraud. Through our comprehensive product suite, Intelligent Decision Studio, lending agencies can effectively distinguish between fraudulent individuals and genuine borrowers. This capability significantly streamlines credit decisions, making the process easier and more reliable.