The increasing complexity of the global financial markets has driven the need for improved decision-making strategies. With a significant amount of data involved in almost all aspects of the business, decision-making has become complicated and time-consuming.
Manually analysing massive datasets can be costly and inaccurate in the current environment. For this reason, the financial sector increasingly relies on AI-backed analytics to make better and faster data-driven decisions. The Mordor Intelligence Report estimates that the demand for ML and AI in fintech alone will reach $26.67 billion by 2026 and will find application in many different areas, including but not limited to data collection and analysis.
Additionally, among the many AI-backed analytics types used by the financial sector today, predictive and prescriptive analytics are two essential tools in the financial decision-making process, allowing companies to identify risks, develop strategies, and optimise their investments.
What is Predictive Analytics?
With the global market for predictive analytics expected to grow to $41.52B in 2028, it is one of the most widely used tools for analysing large amounts of data. Predictive analytics analyses past data to identify patterns, relationships, and trends that can help predict future outcomes using machine learning (ML) and artificial intelligence (AI).
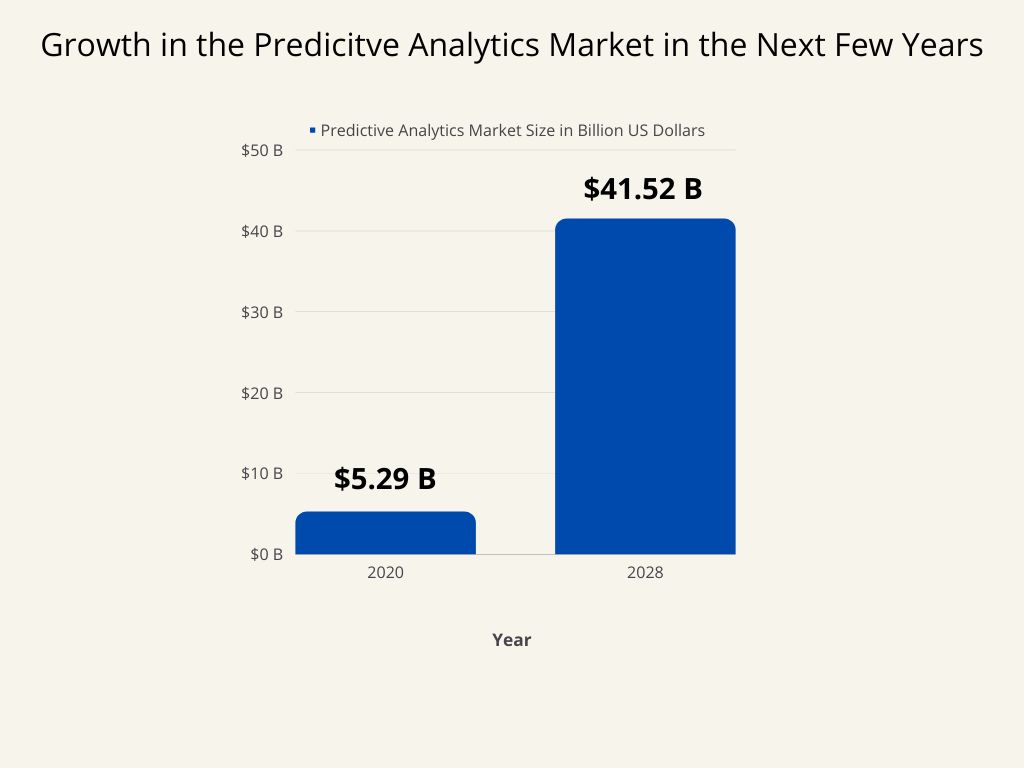
Stay tuned for updates, news on finance & technology
For example, a financial services firm may use predictive analytics to forecast risk levels associated with a customer’s portfolio or to assess the likelihood of loan defaults in the future depending on the past financial behaviour of the customer.
A few of the standard ML and AI techniques used for predictive analytics are regression analysis and random forest, which are also part of Corestrat’s Model.ai, a no-code, enterprise-ready platform that lets you build and deploy predictive models for classifications and regressions with just a few clicks.
Because of recent advances in the speed and accuracy of predictive analytics, financial institutions can broaden the scope of their implementation and apply the technology more broadly across strategic and tactical areas of their business.
What is Prescriptive Analytics?
Prescriptive analytics proposes a plan of action or strategy by considering data regarding potential situations or scenarios, available resources, and past and present performance. It uses analytical techniques like predictive, descriptive, and diagnostic analytics to deliver actionable insights to decision-makers, allowing them to make decisions based on the most statistically sound data and models.
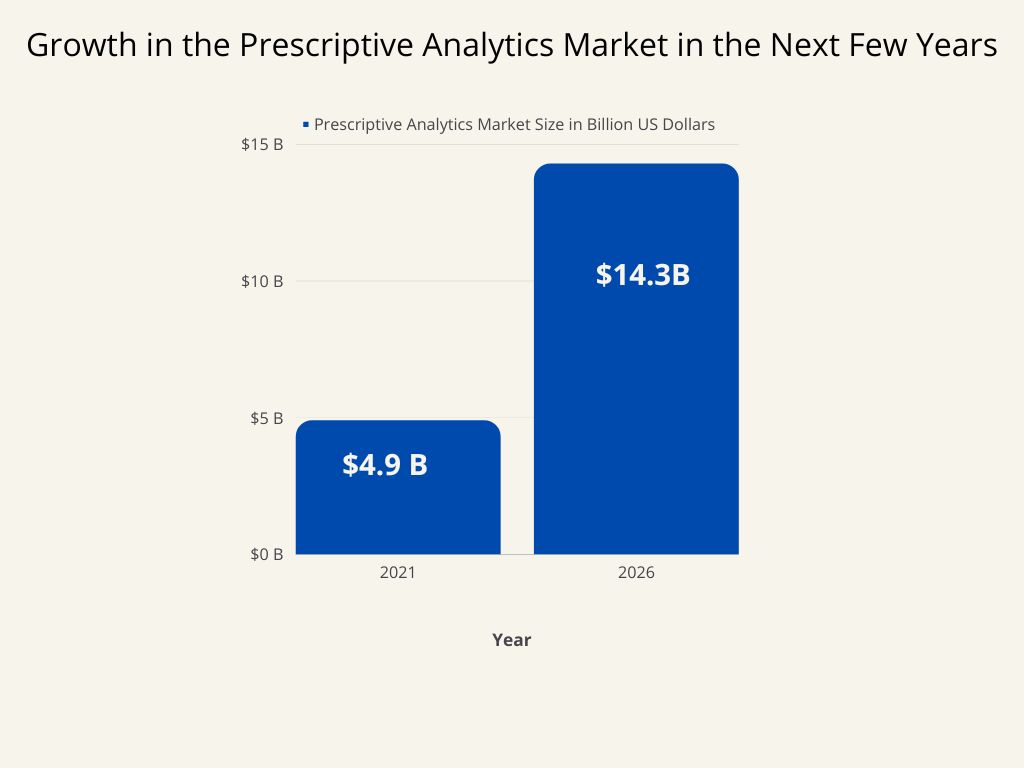
For example, a financial services firm may use prescriptive analytics to determine which investments are most likely to yield the highest returns. Financial institutions can use this type of analytics to identify potential customer segments and choose the best strategies to target them. Banks and other financial institutions use prescriptive analytics to better identify profitable opportunities and make more informed decisions.
A forecast shows the prescriptive analytics market will grow by 24% over the forecast period, from $4.9 billion in 2021 to $14.3 billion by 2026. Machine learning and artificial intelligence (AI) are critical components of prescriptive analytics because they enable the system to learn from data and improve its predictions over time.
Machine learning algorithms are used to find patterns in data and predict what will happen next. Artificial intelligence techniques such as neural networks and decision trees are used to create models that can predict future outcomes based on historical data.
Combination Of Predictive And Prescriptive Analytics In Financial Decision-Making
The combination of predictive and prescriptive analytics is becoming increasingly important for best-in-class financial decision-making. Predictive analytics helps identify potential trends or patterns in the data, while prescriptive analytics helps to determine the best course of action to take in a given situation. By leveraging both forms of analytics, financial firms can make more informed decisions and better assess risk.
These methods are being used in a variety of financial applications, including portfolio management, asset allocation, credit risk assessment, and customer segmentation. For example, a financial services firm may use predictive analytics to forecast the future credit risk of a customer segment and then use prescriptive analytics to determine the best strategies to mitigate risk.
Predictive and prescriptive analytics are also used in the insurance industry to assess risk more accurately and to develop more targeted products. Companies use predictive models to identify potential fraudulent activity and to develop prevention strategies. Insurance firms are also using predictive models to assess customer risk profiles and determine the best products to offer them.
Benefits of Predictive and Prescriptive Analytics in Financial Decision Making
Financial institutions are increasingly using predictive and prescriptive analytics to make better decisions, gaining a competitive advantage as well as increasing margins. The key benefits of using predictive and prescriptive analytics in financial decision-making are:
- Better Decision Making: Predictive analytics enables financial institutions to forecast future events and identify potential risks, whereas prescriptive analytics advises on the best course of action to take. By combining these two, financial institutions can make more informed decisions based on data-driven insights.
- Improved Efficiency: Predictive and prescriptive analytics assist financial institutions in automating decision-making processes, reducing manual workloads, and improving operational efficiency. This can help save time and money associated with manual decision-making processes.
- Increased Revenue: By analysing customer behaviour, identifying customer needs, and tailoring products and services to meet those needs, predictive and prescriptive analytics can assist financial institutions in identifying new revenue opportunities. The net result is increased customer loyalty and customer lifetime value.
- Risk Management: Predictive and prescriptive analytics can help financial institutions manage risk more effectively by identifying potential risks and recommending mitigation strategies. This can help reduce losses and protect the institution’s reputation for all stakeholders.
- Enhanced customer experience: Predictive and prescriptive analytics can help financial institutions better understand their customers and tailor their offerings accordingly. As a result, customers will be more satisfied and loyal when they receive a personalised and engaging experience.
Bottom Line
By using machine learning and AI algorithms to analyse data and provide recommendations, predictive and prescriptive analytics can help financial institutions gain a competitive advantage and provide better services to their customers.
Corestrat, a leading name in providing digital transformation solutions, delivers financial firms with the capabilities to analyse huge amounts of data through our enterprise-ready no-code model builder, Model.ai. Data scientists and non-data professionals can reduce repetitive tasks through machine-learning with Model.ai, enabling them to quickly adopt cutting-edge, AI-powered solutions thus drastically improving informed decision-making.
If this interests you and would like to discuss your business objectives with us, please feel free to fill this form or write to us at [email protected].